What's next for Databricks? The future of acquisitions for the $43B joggernaut
Musings on what is next for Databricks as explored through the acquisitions of startup's past
So, today I start off by acknowledging my blunder in Substack publishing automation which resulted in no Day to Data last week! 10 whole months and that’s never happened! So I figured we’d just skip the week and wait until the following Sunday. There had to be something spooky to end October, so missing a Day to Data was that. Next week will be my month recap for October.
One more interjection from future Gaby. I wrote this piece mid October, ready to post October 22. And in a beautiful turn of events making me feel quite psychic, on October 23, Databricks announced an acquisition of Arcion. The goal of the acquisition is to enable Databricks to provide native solutions to ingest data from various databases into the Databricks Lakehouse Platform. I have not made any changes to the article since writing this pre-acquisition (so Arcion isn’t mentioned in any of my charts or analysis), and while I didn’t predict that a CDC-forward, database consolidation platform would be their next acquisition, I did think Databricks may focus on latency. The company wants their customers to be able to move faster than anyone else, and Arcion aligns with the push towards latency improvements.
This article is a bit beefier in tech and analysis than I typically write, but it’s something I’m working on getting more comfortable with. Thanks for reading!
Today, we’re talking about Databricks — the $43B BEHEMOTH of a company birthday birthed through the open source movement. I could, and just might, write several posts about Databricks, but today I’m taking an angle I’ve been thinking about lately.
We’ll dive into the tech, the people, and what I believe lies in the road ahead, all while I laugh about the time that I had one of the highest Databricks’ spends at my old job as a data scientist.
Built on groundbreaking research
After leaving Iran during his youth, Ali Ghodsi’s family settled in Sweden, where he tinkered with old broken gaming devices. This resulted in him learning how to code at the age of 8. Fast forward, Ghodsi arrived at UC Berkeley in 2009 to spend a year researching machine learning and data processing. While at UC Berkeley, Ghodi and team open sourced Apache Spark, a ground breaking approach to data processing that uses parallelism to achieve never before seen speeds. After its release, Spark set a new record for large-scale sorting of 1 TB of data, performing 3x faster on 10x less hardware than existing state of the art technology in the Daytona Graysort contest. For reference, the previous winner Hadoop had sorted 1.42TB/min. Databricks and Apache Spark sorted 4.27BTB/min. The 2016 winner and reigning champ, Tencent Sort, is sorting 44.8TB/min. Mind. Blowing.
In 2013, Ghodsi cofounded Databricks alongside fellow UC Berkeley researchers in hopes of building a data and machine learning platform that utilized the functionality and speed of Apache Spark to supercharge the future of big data.
Databricks grew and they haven’t stopped
From its humble roots in that Berkeley lab to the $43B company it was valued at earlier in 2023, Databricks has quickly worked its way into enterprises all over the world, across every sector as being the de facto tool to scale data-driven decision making and machine learning capabilities. And they haven’t done it all on their own — they’ve acquired some incredible companies along their journey. I want to dive into Databricks’ acquisitions and what I expect their next bet will be.
The trajectory of their acquisitions fall into three stages:
Enhance data visualization and accessibility
Drive ROI through simplicity for users
Become the AI platform through infrastructure and MLOps (machine learning operations)
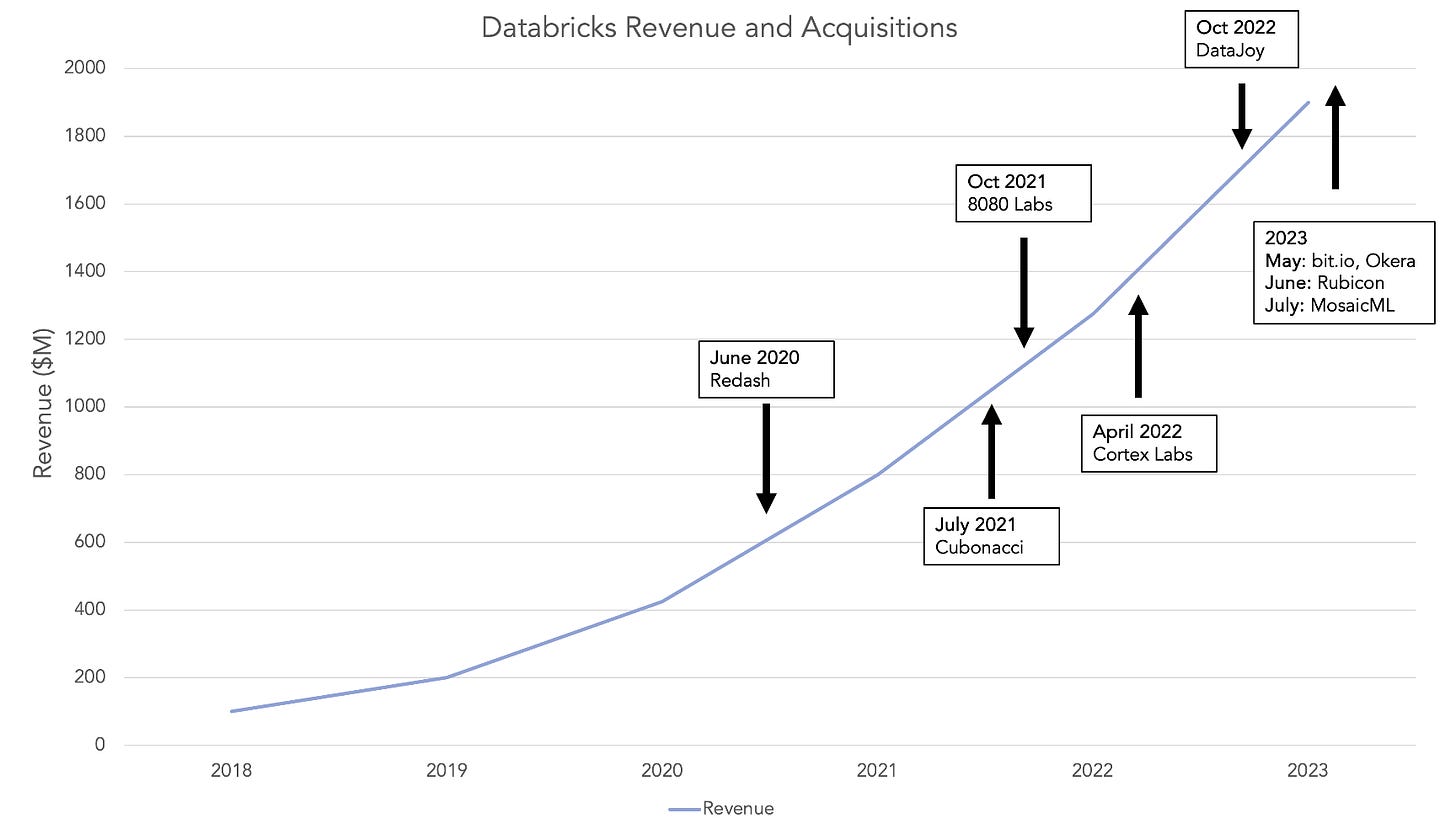
Stage 1: Enhance data visualization and accessibility
Databricks’ first acquisitions were focused on building out their team through acquiring great teams, as well as making their platform (that was born out of researchers doing state of the art data engineering) easy to use for enterprise teams. There was a tangible need as Databricks took off in the early days for the company to focus on making a platform that 1) had incredible UX and 2) scaled the complex research the product was built on top of.
June 2020: Redash helped data scientists and analysts visualize their data and build dashboards around it, with customers like Atlassian, Cloudflare, and Soundcloud. The product had an open-source self-hosted version as well as paid hosted options. Redash enabled Databricks to let
July 2021: Cubonacci, HQ’d in Amsterdam, shared a vision with Databricks to enable data science at scale. The two senior hires are now Staff PMs at Databricks.
Oct 2021: 8080 Labs was a German startup that made bamboolib, a popular UI-based data science tool to enable fast and easy data exploration and transformation with low/no code to expand the accessibility of Databricks lakehouse platform.
Stage 2: Drive ROI through simplicity for users
The product and technology are capturing customer attention. Now, Databricks wanted to keep them and expand the TAM (total addressable market) by continuing to drive down ease of use. Data scientists and engineers were on the platform in troves, but teams that didn’t have the full stack needed to be able to get value from the platform. Databricks’ next stage of acquisitions helped fortify the ROI driving outcomes of using Databricks.
April 2022: Cortex Labs was the developer of Cortex, an open-source platform for deploying, managing, and scaling ML models in production. Cortex enables engineers and data scientists to deploy ML models in production without worrying about DevOps or cloud infra
Oct 2022: DataJoy enabled the simplification of business teams’ ability to be more data driven, removing the need for data engineers to clean, organize, and establish infrastructure. DataJoy focused on revenue insights and performance dashboards for non-technical teams at SaaS companies.
May 2023: bit.io simplifies data management for developers, making set up time just a few seconds.
Stage 3: Become the AI platform through infrastructure and MLOps
It was clear that Databricks was well positioned to take on the future of AI. With their 2023 acquisitions — 3 already and its only October — Databricks is priming itself to be the AI platform of the past, present, and future. Their 2023 acquisitions have been high ticket transactions. Mosaic ML was bought for $1.3B. These acquisitions were clearly selling the right narrative, as Databricks raised their Series I (over $500M) in September, reaching a $43B valuation.
May 2023: Okera is an AI-centric data governance platform to discover unprotected sensitive data and enforce governance of workloads. Okera was founded by the creator of Apache Parquet.
June 2023: Rubicon enables the next generation of storage infrastructure for AI, started by the team behind Dropbox’s incredible storage product
July 2023: Mosaic ML democratizes the development of generative AI models for enterprise, using proprietary data and processes, and tightly integrates into the Lakehouse AI platform
So what’s in the future?
My bet for Databricks’ future acquisitions fall in to one of three categories —
Making LLMs easy — startups that tackle things like drag and drop model development, ways to get behind the “black box” nature of neural networks, and explainability/interpretation tools for LLMs. I suspect they may invest in building out their existing vector database and search capabilities to support model training and development.
Latency — running on Spark is not enough for the modern user. Databricks’ has continued to evolve their tech stack to optimize for speed, but I expect that they’ll make an investment in improving latency across their stack to continue serving customer’s cutting edge needs.
Hardware — Databricks has so much data. They currently manage and deploy cloud infrastructure with their lakehouse to a customer’s cloud, but I think there may be room for Databricks to improve their offerings by investing in hardware to support their evolving data needs.
Thanks for reading.
That’s a wrap for this week! Hope you enjoyed diving into Databricks and their acquisition journey. We might see another Databricks’ acquisition before year end — let’s see if any of my predictions were right!
Love the technical analysis
Well above my pay grade. You should be writing a treatment for Silicon Valley II for Max, rather than only writing term sheets and Day to Data posts.