Today, in the last part of our series on recommendation systems, we will discuss some of the social implications of recommendation systems. We’ll discuss how humans interact with machine-generated recommendations and what it could mean when we leave the deciding up to the data. Catch up on our previous posts starting here:
do we trust machines more than humans?
In a recent episode of Hard Fork, hosts Kevin Roose and Casey Newton discussed a fascinating topic Roose coined called machine drift.
The duo discussed Spotify’s new AI DJ and how they worry a bit about whether it took the joy out of creating a good playlist, finding new music on a blog or from a friend, and the other human parts of music curation. Letting go of some of the autonomy and giving up the typically enjoyable tasks to AI play into this idea of machine drift.
In Futureproof, Roose discusses strategies to maintain autonomy in the ever growing automation age. Recommendation engines, while they do reduce friction while navigating platforms, “pull us back to the middle of the bell curve”. When we are purely looking to recommendations for insight, we aren’t relying as much on our own instincts, scrutinizing our choices, and asking ourselves if these recommendations really match our own preferences.
There's a few different things to think about here — how we trust machine recommendations versus a recommendation coming from a real person, and how we trust machine recommendations versus our own choices and preferences.
trusting recommendations from a machine versus following our own preferences.
Is it a waste of my time to take recommendations from a machine on areas that I have my own strong preferences over? I minimally use recommendations when shopping for clothing as I’ve got a strong idea of what I’m looking for, but when it comes to a new mattress, water bottle, or TV, I’ll let the recommendations on Amazon speak for themselves.
Experiments have found a pattern potentially supporting my feelings — in a Harvard Business Review article, the authors concluded after several experiments that “consumers tend to believe AI is more competent at making recommendations when they are seeking functional or practical offerings and humans when they are more interested in an offering’s experiential or sensory features”. Their findings suggest that humans are more trusted when it comes to recommending something like chocolate cake recipes versus machines when it comes trusting AI when looking at recommendations for a new computer to buy.
trusting machines versus humans.
A set of researchers at Penn State investigated whether people trust machines or humans with their personal information. The experiments concluded that when it comes to “private information and access to financial data, people tend to trust machines more than people”. I wouldn’t tell a stranger my address, but I do give it up freely every time I order something online.
An area to emphasize in research is the language used to describe artificial intelligence does affect how humans respond. For example, researchers found that when using language like “augmented intelligence that enhances and supports humans” fares similarly to human recommendations.
Look at StitchFix, the styling platform, that embeds data science into its recommendations alongside a human stylist. Depending on the domain, suggesting that the machine isn’t doing all of the work but rather helping a human make a recommendation provides a sense of humanity to the process. If you don’t like your outfit, you can’t blame it entirely on the machine!
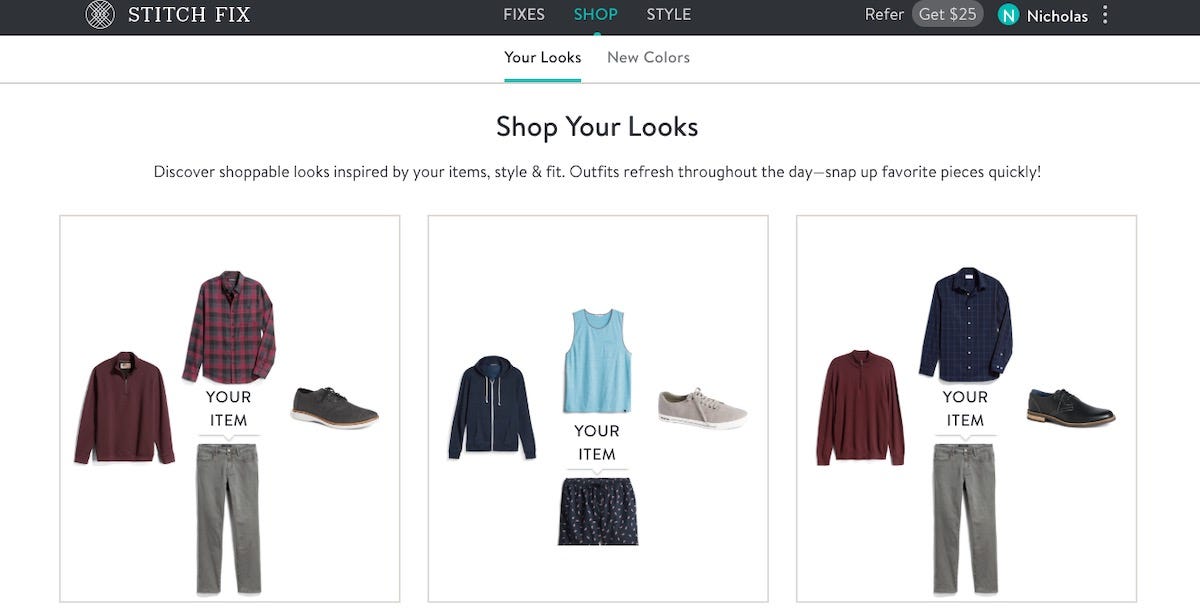
my take.
While researching today’s article, I’ve gotten more interested in this intersection of recommendation systems and the everyday user. How can we work alongside recommendation systems to find the next movie we love, the perfect wedding guest dress, or even who we’ll go on our next date with?
There is a case that the overuse of recommendation systems should worry us. If we only interact with recommendations, where do we exercise our own preferences? Can recommendation systems be skewed by paid products and brand deals? Do recommendation systems manipulate our own true preferences?
To be frank, these things don’t worry me because I’m aware of the role that recommendation systems play in my day to day. I’ve implemented a few simple practices like:
Setting time limits on Instagram and Youtube (apps that I feel can overwhelm me with recommendations, and a lot of the times, they are ones I don’t truly like)
Continue doing the things I’ve always enjoyed that have since seen replacements by recommendation systems or artificial intelligence — like making playlists and finding new recipes
I do still reap the benefits of recommendation systems. GoodReads has notified me of some of my favorite books I’ve read this year and Getir somehow knows the snacks I want before I crave them. For me, it’s the balance of enjoying the benefits of machine learning and data science, while being aware of the impact algorithms have on my everyday.
Thanks for reading this week’s Day to Data. We’re almost 16 weeks old! That’s pretty epic. Glad to have you around. Follow us on Twitter @day__to__data to stay updated, or reach out with ideas and questions.